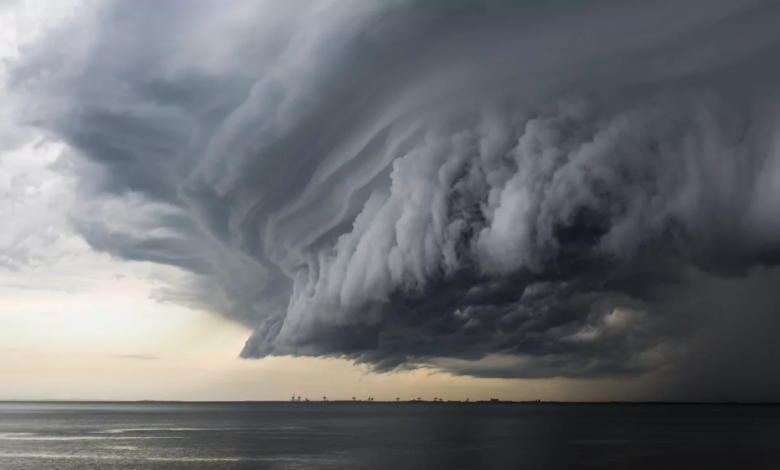
Artificial intelligence (AI) has enormous value but capturing the full benefits of AI means facing and handling its potential pitfalls. The same sophisticated systems used to discover novel drugs, screen diseases, tackle climate change, conserve wildlife and protect biodiversity can also yield biased algorithms that cause harm and technologies that threaten security, privacy and even human existence.
Here’s a closer look at 10 dangers of AI and actionable risk management strategies. Many of the AI risks listed here can be mitigated, but AI experts, developers, enterprises and governments must still grapple with them.
1. Bias
Humans are innately biased, and the AI we develop can reflect our biases. These systems inadvertently learn biases that might be present in the training data and exhibited in the machine learning (ML) algorithms and deep learning models that underpin AI development. Those learned biases might be perpetuated during the deployment of AI, resulting in skewed outcomes.
AI bias can have unintended consequences with potentially harmful outcomes. Examples include applicant tracking systems discriminating against gender, healthcare diagnostics systems returning lower accuracy results for historically underserved populations, and predictive policing tools disproportionately targeting systemically marginalized communities, among others.
Take action:
- Create practices that promote fairness, such as including representative training data sets, forming diverse development teams, integrating fairness metrics, and incorporating human oversight through AI ethics review boards or committees.
- Put bias mitigation processes in place across the AI lifecycle. This involves choosing the correct learning model, conducting data processing mindfully and monitoring real-world performance.
- Look into AI fairness tools, such as IBM’s open source AI Fairness 360 toolkit.
2. Cybersecurity threats
Bad actors can exploit AI to launch cyberattacks. They manipulate AI tools to clone voices, generate fake identities and create convincing phishing emails—all with the intent to scam, hack, steal a person’s identity or compromise their privacy and security.
And while organizations are taking advantage of technological advancements such as generative AI, only 24% of gen AI initiatives are secured. This lack of security threatens to expose data and AI models to breaches, the global average cost of which is a whopping USD 4.88 million in 2024.
Take action:
Here are some of the ways enterprises can secure their AI pipeline, as recommended by the IBM Institute for Business Value (IBM IBV):
- Outline an AI safety and security strategy.
- Search for security gaps in AI environments through risk assessment and threat modeling.
- Safeguard AI training data and adopt a secure-by-design approach to enable safe implementation and development of AI technologies.
- Assess model vulnerabilities using adversarial testing.
- Invest in cyber response training to level up awareness, preparedness and security in your organization.
3. Data privacy issues
Large language models (LLMs) are the underlying AI models for many generative AI applications, such as virtual assistants and conversational AI chatbots. As their name implies, these language models require an immense volume of training data.
But the data that helps train LLMs is usually sourced by web crawlers scraping and collecting information from websites. This data is often obtained without users’ consent and might contain personally identifiable information (PII). Other AI systems that deliver tailored customer experiences might collect personal data, too.
Take action:
- Inform consumers about data collection practices for AI systems: when data is gathered, what (if any) PII is included, and how data is stored and used.
- Give them the choice to opt out of the data collection process.
4. Environmental harms
AI relies on energy-intensive computations with a significant carbon footprint. Training algorithms on large data sets and running complex models require vast amounts of energy, contributing to increased carbon emissions. One study estimates that training a single natural language processing model emits over 600,000 pounds of carbon dioxide; nearly 5 times the average emissions of a car over its lifetime.1
Water consumption is another concern. Many AI applications run on servers in data centers, which generate considerable heat and need large volumes of water for cooling. A study found that training GPT-3 models in Microsoft’s US data centers consumes 5.4 million liters of water, and handling 10 to 50 prompts uses roughly 500 milliliters, which is equivalent to a standard water bottle.2
Take action:
- Consider data centers and AI providers that are powered by renewable energy.
- Choose energy-efficient AI models or frameworks.
- Train on less data and simplify model architecture.
- Reuse existing models and take advantage of transfer learning, which employs pretrained models to improve performance on related tasks or data sets.
- Consider a serverless architecture and hardware optimized for AI workloads.
5. Existential risks
In March 2023, just 4 months after OpenAI introduced ChatGPT, an open letter from tech leaders called for an immediate 6-month pause on “the training of AI systems more powerful than GPT-4.”3 Two months later, Geoffrey Hinton, known as one of the “godfathers of AI,” warned that AI’s rapid evolution might soon surpass human intelligence.4 Another statement from AI scientists, computer science experts and other notable figures followed, urging measures to mitigate the risk of extinction from AI, equating it to risks posed by nuclear war and pandemics.5
While these existential dangers are often seen as less immediate compared to other AI risks, they remain significant. Strong AI or artificial general intelligence, is a theoretical machine with human-like intelligence, while artificial superintelligence refers to a hypothetical advanced AI system that transcends human intelligence.
Take action:
Although strong AI and superintelligent AI might seem like science fiction, organizations can get ready for these technologies:
- Stay updated on AI research.
- Build a solid tech stack and remain open to experimenting with the latest AI tools.
- Strengthen AI teams’ skills to facilitate the adoption of emerging technologies.
6. Intellectual property infringement
Generative AI has become a deft mimic of creatives, generating images that capture an artist’s form, music that echoes a singer’s voice or essays and poems akin to a writer’s style. Yet, a major question arises: Who owns the copyright to AI-generated content, whether fully generated by AI or created with its assistance?
Intellectual property (IP) issues involving AI-generated works are still developing, and the ambiguity surrounding ownership presents challenges for businesses.
Take action:
- Implement checks to comply with laws regarding licensed works that might be used to train AI models.
- Exercise caution when feeding data into algorithms to avoid exposing your company’s IP or the IP-protected information of others.
- Monitor AI model outputs for content that might expose your organization’s IP or infringe on the IP rights of others.
7. Job losses
AI is expected to disrupt the job market, inciting fears that AI-powered automation will displace workers. According to a World Economic Forum report, nearly half of the surveyed organizations expect AI to create new jobs, while almost a quarter see it as a cause of job losses.6
While AI drives growth in roles such as machine learning specialists, robotics engineers and digital transformation specialists, it is also prompting the decline of positions in other fields. These include clerical, secretarial, data entry and customer service roles, to name a few. The best way to mitigate these losses is by adopting a proactive approach that considers how employees can use AI tools to enhance their work; focusing on augmentation rather than replacement.
Take action:
Reskilling and upskilling employees to use AI effectively is essential in the short-term. However, the IBM IBV recommends a long-term, three-pronged approach:
- Transform conventional business and operating models, job roles, organizational structures and other processes to reflect the evolving nature of work.
- Establish human-machine partnerships that enhance decision-making, problem-solving and value creation.
- Invest in technology that enables employees to focus on higher-value tasks and drives revenue growth.
8. Lack of accountability
One of the more uncertain and evolving risks of AI is its lack of accountability. Who is responsible when an AI system goes wrong? Who is held liable in the aftermath of an AI tool’s damaging decisions?
These questions are front and center in cases of fatal crashes and hazardous collisions involving self-driving cars and wrongful arrests based on facial recognition systems. While these issues are still being worked out by policymakers and regulatory agencies, enterprises can incorporate accountability into their AI governance strategy for better AI.
Take action:
- Keep readily accessible audit trails and logs to facilitate reviews of an AI system’s behaviors and decisions.
- Maintain detailed records of human decisions made during the AI design, development, testing and deployment processes so they can be tracked and traced when needed.
- Consider using existing frameworks and guidelines that build accountability into AI, such as the European Commission’s Ethics Guidelines for Trustworthy AI,7 the OECD’s AI Principles,8 the NIST AI Risk Management Framework,9 and the US Government Accountability Office’s AI accountability framework.10
9. Lack of explainability and transparency
AI algorithms and models are often perceived as black boxes whose internal mechanisms and decision-making processes are a mystery, even to AI researchers who work closely with the technology. The complexity of AI systems poses challenges when it comes to understanding why they came to a certain conclusion and interpreting how they arrived at a particular prediction.
This opaqueness and incomprehensibility erode trust and obscure the potential dangers of AI, making it difficult to take proactive measures against them.
“If we don’t have that trust in those models, we can’t really get the benefit of that AI in enterprises,” said Kush Varshney, distinguished research scientist and senior manager at IBM Research® in an IBM AI Academy video on trust, transparency and governance in AI.
Take action:
- Adopt explainable AI techniques. Some examples include continuous model evaluation, Local Interpretable Model-Agnostic Explanations (LIME) to help explain the prediction of classifiers by a machine learning algorithm and Deep Learning Important FeaTures (DeepLIFT) to show a traceable link and dependencies between neurons in a neural network.
- AI governance is again valuable here, with audit and review teams that assess the interpretability of AI results and set explainability standards.
10. Misinformation and manipulation
As with cyberattacks, malicious actors exploit AI technologies to spread misinformation and disinformation, influencing and manipulating people’s decisions and actions. For example, AI-generated robocalls imitating President Joe Biden’s voice were made to discourage multiple American voters from going to the polls.11
In addition to election-related disinformation, AI can generate deepfakes, which are images or videos altered to misrepresent someone as saying or doing something they never did. These deepfakes can spread through social media, amplifying disinformation, damaging reputations and harassing or extorting victims.
AI hallucinations also contribute to misinformation. These inaccurate yet plausible outputs range from minor factual inaccuracies to fabricated information that can cause harm.
Take action:
- Educate users and employees on how to spot misinformation and disinformation.
- Verify the authenticity and veracity of information before acting on it.
- Use high-quality training data, rigorously test AI models, and continually evaluate and refine them.
- Rely on human oversight to review and validate the accuracy of AI outputs.
- Stay updated on the latest research to detect and combat deepfakes, AI hallucinations and other forms of misinformation and disinformation.
Make AI governance an enterprise priority
AI holds much promise, but it also comes with potential perils. Understanding AI’s potential risks and taking proactive steps to minimize them can give enterprises a competitive edge.
With IBM® watsonx.governance™, organizations can direct, manage and monitor AI activities in one integrated platform. IBM watsonx.governance can govern AI models from any vendor, evaluate model accuracy and monitor fairness, bias and other metrics.
All links reside outside ibm.com
1 Energy and Policy Considerations for Deep Learning in NLP, arXiv, 5 June 2019.
2 Making AI Less “Thirsty”: Uncovering and Addressing the Secret Water Footprint of AI Models, arXiv, 29 October 2023.
3 Pause Giant AI Experiments: An Open Letter, Future of Life Institute, 22 March 2023.
4 AI ‘godfather’ Geoffrey Hinton warns of dangers as he quits Google, BBC, 2 May 2023.
5 Statement on AI Risk, Center for AI Safety, Accessed 25 August 2024.
6 Future of Jobs Report 2023, World Economic Forum, May 2023.
7 Ethics guidelines for trustworthy AI, European Commission, 8 April 2019.
8 OECD AI Principles overview, OECD.AI, May 2024.
9 AI Risk Management Framework, NIST, 26 January 2023.
10 Artificial Intelligence: An Accountability Framework for Federal Agencies and Other Entities, US Government Accountability Office, 30 June 2021.
11 New Hampshire investigating fake Biden robocall meant to discourage voters ahead of primary, AP News, 23 January 2024.
Was this article helpful?
YesNo
Source link